Hilke Schellmann exposes the flaws in AI that control your next job offer
Dec 23, 2024
10 mins
US Editor at Welcome to the Jungle
Hilke Schellmann, an Emmy-winning investigative journalist and assistant professor of journalism at New York University, brings her expertise to the critical examination of AI hiring tools in her latest book, The Algorithm: How AI Decides Who Gets Hired, Monitored, Promoted, and Fired and Why We Need to Fight Back Now. Known for her work on technology and ethics, Schellmann uncovers how these tools—designed to streamline hiring—can often introduce or exacerbate biases.
Through her investigative research, Schellmann highlights how AI hiring tools, despite being marketed as efficient and objective, frequently make flawed decisions that impact countless job seekers. She has even tested these technologies herself, revealing unsettling patterns of inconsistency and bias in the algorithms used by some of the largest corporations. Her insights raise serious questions about fairness, transparency, and accountability in a landscape where job opportunities increasingly hinge on automated processes.
As the use of AI hiring tools in recruitment is increasing, Schellmann’s findings push us to think critically about the ethical implications of letting algorithms shape careers and lives.
How are you seeing AI impact the hiring process today, and what are some ways it’s being used?
Many people don’t realize just how extensive AI’s role has become in hiring. Research and survey data show that nearly all Fortune 500 companies—over 90%—use AI or algorithms somewhere in the hiring funnel.
The sheer volume of applications companies receive is why they’re turning to AI. For example, Google receives about 3 million applications each year, and IBM around 5 million. Goldman Sachs reported over 200,000 applications for their summer internship program a few summers back. Companies are drowning in resumes, and they are looking for technical solutions.
If you’ve ever used any large job platform like LinkedIn or ZipRecruiter, you’ve likely interacted with AI. They sort, filter, and match profiles to roles—streamlining the process for recruiters by automatically ranking applications based on certain criteria to manage the vast volume of applications.
Beyond resume screening, we’re also seeing AI-based one-way video interviews where candidates record themselves on their phone or desktop computer, answering pre-recorded questions without a human on the other side. AI then analyzes these responses. Similar AI-driven processes are also being used with phone or audio interviews.
Additionally, algorithms are used in various other assessments, such as coding and capability tests. AI is even used in background checks and can scrape candidates’ X or LinkedIn feeds to run personality and job compatibility profiles.
This extensive usage of AI and algorithms is largely not visible to job seekers. Every applicant we’ve spoken to assumed that humans were watching the one-way video interviews that they recorded of themselves, and while that may sometimes be true, it isn’t always the case.
We also see that ATS (Applicant Tracking) systems now integrate AI into their processes, but we don’t really know for sure—at least not in the US — which functions companies turn on or off. I have not heard of a country that requires companies to publicly disclose or inform a government agency if these AI tools are active. We simply don’t have that transparency. All we know is that these tools exist, and companies, overwhelmed by the sheer volume of resumes, turn to these and similar technologies to manage the load.
I heard you conducted some experiments by posing as a job seeker to test these AI tools yourself. Could you share some of those experiences with us?
Goodness … I took so many video interviews. I wanted to test the technology and really know how it felt to be a job seeker using these tools. But, I also wanted to understand the edge cases and see how these systems would treat me in different scenarios.
I was honestly a bit stunned when I completed a video interview that used AI-based analysis, and I only repeated three words. I kept saying, “I love teamwork,” when answering every question, and yet I still received a pretty high score.
Another tool that I tested was built and marketed to companies in the West hiring call center employees in the Global South, where one of the most important criteria was how well the candidate speaks English. So, I bought myself a license and set up a job to interview myself. When I spoke English and tried to answer the questions accurately, I received an 8.5 out of 9 English-competent score.
And then I thought about people who have an accent or perhaps a speech disability—how would they be treated by these systems? Would the system accurately assess or misinterpret how well someone speaks English? I have a slight accent [in English], so I wondered how I could test this.
These companies had told me that if someone has a speech disability or strong accent that the tool couldn’t accurately decipher, the company using the tool would receive an error message prompting them to request an accommodation, potentially leading to a human interviewer.
So, I spoke in German and read a Wikipedia entry about psychometrics—nothing job-related, no mention of teamwork or anything like that. I just read this Wikipedia entry in German. I was surprised to receive a 6 out of 9 English-competent score. I repeated this test with another tool, MyInterview, and saw similar results.
The tool generated a transcription, which, because I spoke in German, turned out gibberish. Still, I received a 73% match rate to the job, indicating I was considered qualified, even though nothing I said “made sense.”
I know my tests aren’t exactly comprehensive or academic, but I think they reveal something important: these systems can be tricked surprisingly easily, and they make basic mistakes. If all it takes to mislead the system is speaking in a different language, what is it actually analyzing or inferring? Can it really assess English proficiency? This raises some fundamental questions about how well these tools work and whether they’re delivering on their intended purpose.
Did you ever try the video game tests?
Yes! So, some companies ask candidates to play simple video games to assess skills and personality traits, allegedly drawing on psychological and neuroscience research. The aesthetics of the games are often basic, more like something from 90s Pac-Man. One game I played had me pumping up a balloon and “earning” money with each pump. I had to “cash out” before the balloon burst to keep my earnings. The idea is to measure risk-taking, but it’s unclear if risk-taking in a video game translates to actually measuring real-world risk-taking.
There’s also the issue of calibration: these tools are often based on how people already in the job play the game. But, are these games really testing job-relevant skills, or just qualities that current employees coincidentally have in common that may have nothing to do with the job?
Then there’s the question of accessibility. In one game, I had to hit the spacebar as fast as possible. I wondered what that tool was testing—and, more importantly, how this would impact someone with a motor disability. They’d be at a disadvantage not because they may lack job-relevant skills but simply due to the nature of the game. Would they end up being unfairly screened out or discriminated against just because they physically can’t hit the spacebar as quickly as others, even though they might be able to do the advertised job? This raises significant concerns about fairness and inclusivity.
There’s also a fundamental limitation with personality tests in general. Yes, our personalities are somewhat stable, but as humans, we have the ability to adapt and even overcome certain traits. For example, someone might be naturally shy or struggle with conscientiousness, but they can learn methods to show up on time, meet deadlines, and push past these tendencies. So, these tools may not truly reflect a person’s capabilities and skills but rather capture a single snapshot in time. We as humans actually have the potential to push ourselves beyond our innate traits.
Have you tried to scrape your social media with these tools?
I used my own LinkedIn and X feeds with a few tools designed to “find out who I really am”—essentially, to analyze my personality based on my LinkedIn or X posts. What’s striking is that this can be done without a candidate’s consent.
These tools claim they can extract personality traits like conscientiousness or agreeableness from the written language in social media posts, LinkedIn profiles, and even uploaded resumes or other authored texts. To explore this, I asked Tomas Chamorro-Premuzic, an industrial-organizational psychologist and an AI enthusiast,—to test these tools with me using our own LinkedIn and Twitter profiles to see how they analyzed our traits.
What struck us was that, although these tools are marketed as identifying consistent personality traits, they provided wildly different personality assessments across platforms, even when the same tool analyzed different feeds of ours. One tool, for instance, analyzed both our LinkedIn and X profiles, yet it presented us as having almost opposite personalities on each platform. Psychology suggests that personality is fairly consistent, but can shift slowly over time, typically over months or years, not in a matter of minutes. Clearly, these tools weren’t delivering as promised.
We touched on things like speech disabilities, but did you notice any other types of bias?
I’ve talked to several employment lawyers, psychologists, and others who are often brought in to evaluate how these tools really work. In one screening tool, an employment lawyer discovered that if your resume included the word “baseball,” you scored a few points, but if you had the word “softball” on your resume, you got some points deducted—an obvious indicator of possible gender discrimination. Another expert also found similar biases.
One lawyer was brought in to evaluate an AI-based tool in the pilot phase, testing it in multiple ways while the vendor created various models. However, none of the approaches worked—the tool consistently discriminated against women by not passing the 4/5 rule [a US Equal Employment Opportunity Commission guideline requiring selection rates for both genders and racial groups to be at least 80% of those for the majority group], which is essentially the bare minimum for compliance.
Dr. John Scott discovered that in one of the resume screening tools, the word “Canada” was a predictor of success, which could indicate discrimination based on national origin. In another tool, Ken Willner, an employment lawyer, found that terms like “Africa” and “African-American” were being used as factors.
Often, there’s little scrutiny for other biases—companies rarely examine disabilities or other criteria. I haven’t seen any checks for age, either. These are all critical factors that could be embedded in a tool and cause harm, but developers often don’t find the problem because few people investigate, perhaps because some vendors might uncover something they’d rather not know.
I’ve spoken with some employment lawyers who focus on AI, and one of them remarked that “none of these AI tools are ready for prime time.” They consistently find issues, which should really give us pause.
“Rather than addressing core role requirements … we’re often automating existing biases.”
Why do so many of these tools miss the mark when it comes to predicting job success?
I think there’s a fundamental problem with many of these tools, especially when they attempt to predict the future—like whether someone will be a successful employee. That’s really hard to predict because there can be dozens of factors - some known and some not yet known. And many managers and HR leaders have a hard time defining what success looks like in a given role.
Plus most AI tools in hiring aren’t focused solely on job-specific criteria. Instead, these systems pull vast amounts of data, including proxies that can be biased. This is problematic because, rather than addressing core role requirements, including skills and capabilities, we’re often automating existing biases.
“Biased proxies” largely stem from non-diverse training data. If a tool is built on resumes from people currently in a job and the company is male-dominated, the tool may pick up on words associated with male employees. That could explain why “baseball” was flagged as a significant keyword—it likely identified baseball as a common word among resumes of successful employees. In contrast, “softball” might appear less frequently on resumes of mostly male employees, leading the system to inadvertently favor one over the other.
Take resumes, if we build AI to rank resumes, does it genuinely improve predictions of who will be successful? We don’t really know. What we do know is that this approach sometimes introduces bias, which goes against the ideal of a merit-based system.
Of course, there is also significant human bias, so I’m not saying we should just go back to human hiring, but we shouldn’t just exchange one biased system with another.
“Millions of people are being assessed with these tools, yet we still don’t have a reliable sense of whether they actually work as intended.”
So if they are so flawed, why are companies still using them?
Everyone includes more or less the same keywords in resumes. This makes it difficult to identify unique qualities, so companies turn to AI tools, hoping they’ll reveal something deeper.
I think many companies are drawn to the idea of hiring for personality, especially post-pandemic. They want employees who not only possess necessary skills, like a software developer knowing Python, but who also show qualities like adaptability and a willingness to learn. This way, when the next programming language or tool emerges, they already have someone who’s agile and ready to upskill on their own.
However, these qualities are difficult to deduce from a resume, making it tempting to use tools that seem like they can look “under the hood” and reveal essential traits about a candidate. But I have found that these tools often overpromise and underdeliver.
Tools like MyInterview are widely used, and when I last checked, they’d stated on their website that they have conducted over five million interviews on their platform. Millions of people are being assessed with these tools, yet we still don’t have a reliable sense of whether they actually work as intended.
But companies continue to use them—they’re readily available on the market, with sleek websites and top-notch marketing language that make it all feel convincing, almost as if it must be true that my personality would show up in the words I write. That’s problematic and even a bit frightening.
So, why all the lack of transparency? Why does it take an investigative journalist to reveal that it doesn’t actually work as advertised?
That is a very good question. I’m not saying that all of them don’t work at all, but I was surprised how many of the tools are flawed. There were a lot of problems in a lot of tools.
An issue I have identified is the pressure from venture capital backing: Many vendors need to deliver returns quickly, so they rush products to market, often without fully validating the science behind them. I’ve spoken with companies, and I would love to run longitudinal studies to see if these tools actually work—comparing AI-based hiring with traditional methods or checking if predictions made today hold true years down the line. That would be invaluable, but so far, no one has agreed to do this with me.
“It’s worth asking: “Can I trick it?” “How was it built?” and “What assumptions are baked into it?””
I’d like to get your thoughts on the core responsibilities that companies should uphold when using these AI systems and platforms.
I believe companies have a responsibility to thoroughly evaluate these AI systems to ensure they don’t discriminate and are genuinely effective, not just pick people at random. We’ve seen issues with some tools, such as video game-based assessments that claim to follow the 4/5 rule to avoid race and gender bias. But do they actually identify the most qualified candidates? That’s uncertain.
I would encourage HR professionals and hiring managers to try similar small tests themselves. When evaluating software, it’s worth asking: “Can I trick it?” “How was it built?” and “What assumptions are baked into it?” These tests can help uncover some of the limitations of these tools.
Additionally, transparency around technical reports is lacking. These reports should detail the system’s setup and tests, but I’ve seen some concerning patterns in the few reports available. For instance, some studies rely on small samples, like college students in their 20s, which raises questions. Are the results generalizable to older candidates or those without a college background? Likely not. As a minimum standard, these technical reports should be made public—but that level of transparency is still missing.
Photo: Yuvraj Khanna for Welcome to the Jungle
Follow Welcome to the Jungle on Facebook, LinkedIn, and Instagram and subscribe to our newsletter to get our latest articles every week!
More inspiration: Follow hiring trends
How AI coaching can give young job hunters an edge
Considering a career coach but don't have the resources to pay for one? AI might be able to help.
Dec 12, 2024
Are internships replacing entry-level jobs?
If an entry-level role requires years of experience, young job hunters are already at a disadvantage …
Mar 06, 2024
Swipe right for success: Using dating apps to job hunt and network
Genius networking move or master manipulation?
Jan 30, 2024
Job search in 2024: A candid look at the US job market
Are layoffs coming to an end? Will you finally land a job in your dream industry? Let’s see what the market has in store …
Jan 22, 2024
CEO seeks organized Taurus: China’s crazy recruitment criteria
If you’re not astrologically compatible, the job is not for you.
Dec 14, 2023
The newsletter that does the job
Want to keep up with the latest articles? Twice a week you can receive stories, jobs, and tips in your inbox.
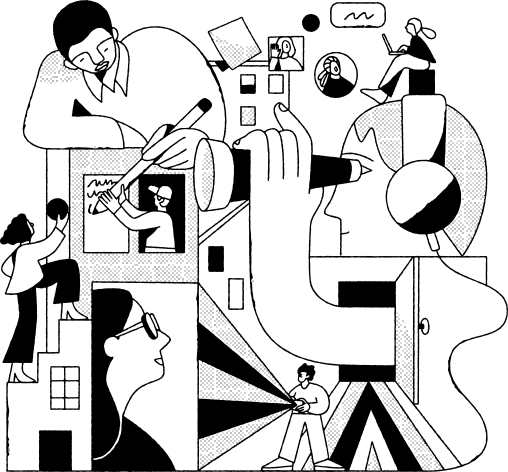
Looking for your next job?
Over 200,000 people have found a job with Welcome to the Jungle.
Explore jobs