What if your recruiter were an algorithm?
Jul 02, 2019
7 mins
Two different sides emerge when the subject of artificial intelligence (AI) arises. One side thinks that these technologies need to be developed cautiously so that we do not find ourselves in some sort of imminent apocalypse à la The Matrix or Terminator. The other doesn’t give any credence to this perspective and believes that we have nothing to fear from the latest technologies, which allow us to delegate the most rote tasks to machines. And the latter point of view seems to touch every sector of business.
Like everything else, the field of digitalized recruiting is expanding, despite the employment process being linked, in large part, to emotional skills via humans rather than pragmatism through robots. In fact, several companies have made it their main business to promote efficient technology-based solutions for recruiting, finding the perfect person to fill a position, or to help narrow down choices based on résumés.
These solutions are tempting for HR departments because poorly calculated hiring can be costly to companies. According to Tilkee’s latest edition of its barometer of recruiter’s habits, a recruiter currently spends an average of 34 seconds reading a CV—21% less time than they did in 2017—which leads us to wonder just what an algorithm can do in 34 seconds.
How does the technology work?
Merriam-Webster defines an algorithm as a procedure for solving a mathematical problem in a finite number of steps that frequently involves repetition of an operation. In other words, we have to imagine a small, virtual robot that is able to apply, at phenomenal speed, the rules one human has programmed in order to carry out a task that would have taken another human much longer to complete. We then add the “machine learning” dimension to this definition and, more broadly, that of AI. From this point, our small robot can now draw from the experience of its work to create newer rules for advancing further and more quickly, just as humans do, only at a much more efficient level. Here, we’ll be talking about the predictive analytics used in job recruiting, where algorithms are used to predict the future performance of employees in their new companies, and we’ll also be distinguishing between sourcing, filtering, and matching algorithms.
Sourcing algorithms
These are configured to search the web for ideal candidates for any given job. They operate in the same way as dating apps: The company lists the skills they are looking for, and then the software uses an algorithm to analyze thousands of online résumés via LinkedIn or public databases. They isolate the most compatible ones and send them to the HR department. All HR needs to do now is contact the people on the shortlist. More and more solutions like this are appearing on the market, such as Yatedo Talent, which promotes itself as the “Google of recruiting.”
Filtering algorithms
Once the maximum number of résumés has been collected (by a sourcing algorithm or by HR), it’s time for the filtering algorithm to do its job. As the name implies, they act as an initial filter. They use a matching hypothesis as the first filter and only send the most qualified profiles to the person in charge of recruiting. Under these circumstances, the algorithm not only analyzes information on the résumés but also deciphers the semantics used by extrapolating the turns of phrases and words, the goal being to delve deeper into the analysis of the candidates.
AssessFirst, a company that offers predictive recruitment solutions, takes it all a step further: Based on three behavioral criteria they identify, the AI system can determine the degree of compatibility between a candidate and a future supervisor.
Matching algorithms
Sourcing and filtering algorithms were created to help recruiting services do their job, but matching-algorithm platforms, such as ZipRecruiter, function as a search engine for people looking for jobs. Applicants post their résumé, which is then parsed using predictive analysis to find the jobs that best correspond to the skills cited on their CVs.
ZipRecruiter proposes open job positions via an algorithm, and the applicant can choose from the various companies. But the companies are also involved, as they will receive shortlisted candidates through the same algorithm—enhancing the classic hiring process.
The advantages of using AI
Better responsiveness for applicants
Tilkee’s barometer also states that, on average, a job recruiter takes 44 hours to contact a candidate—a rather easy record to beat for software that can process data at phenomenal speed. This quicker means of responsiveness is a key added value for companies—at least, when talking about initial contact. With this technology, a pile of résumés can be processed to perfection in just a few seconds, while simultaneously reducing the margin for error.
The range of possibilities
During the job-search stage, it is often difficult to visualize the actual job. It is simpler to take the easy way out and limit ourselves to the positions we feel are more in line with what we want and our skills. A matching algorithm can be beneficial for applicants, in that by analyzing their résumés at a deeper level, AI can propose jobs they would not have considered and thus open up the range of possibilities. Ultimately, the job offers choose the best profiles, not the other way around.
Higher-quality interviews
For businesses, algorithms can provide information above and beyond what someone has put on their résumé. We talked about semantic analysis earlier, which is a difficult task for a person to carry out if they are not trained—and even if they are trained, the margin for error would be too great. In contrast, an algorithm becomes quicker and more efficient after each résumé is analyzed.
Data that can be extracted using semantic tools can provide more information about a candidate’s personality and their experience, depending on their use of wording and technical terms. On the same basis, AI can easily identify issues with dates or locations that a human might not detect. This makes it easier for a company to organize the face-to-face interview and prepare more-focused questions for the applicant. Overall, the first contact will run more smoothly.
An answer to discrimination?
At first glance, trusting a machine to do the initial sorting of applicants seems reassuring: A machine is not racist by nature. It analyzes data, pure and simple. It checks boxes and sends a positive or negative response. This should be enough to reassure everyone involved in recruitment, don’t you think? Actually, it’s not that simple, and everything is not all fine and dandy in the world of predictive analytics.
Why algorithms are still far from infallible
Amazon’s discriminatory algorithm
Employment discrimination, even when machines do the essential filtering work, is still present. Although an algorithm by nature is devoid of thoughts, stereotypes, and prejudices, the fact remains that its configuration is done by human hands. In 2014, Amazon started looking into whether AI could help it sort through people applying to work at the company. By the following year, it had become clear that the algorithm was only approving résumés that had been submitted by men. This unexpected result was explained later by the fact that the system was looking at applications from the previous 10 years, a period during which men were dominating the tech industry, so the mathematical calculations drew the conclusion that men were more appropriate for this type of position.
The error had nothing to do with any bad intention on the part of the person who configured the algorithm, but it did highlight the difficulty in building a perfect algorithm (and blindly trusting it). In 2017, Amazon scrapped the project and returned to traditional hiring methods, putting off the use of predictive analytics for recruiting to a later time.
The imitation effect
Predictive analytics discriminating against job applicants according to their gender is not an across-the-board problem; it was specific to Amazon and the algorithm they built. The most common error in AI, which is not unique to the world of recruitment, is the imitation effect.
Generally, to configure an algorithm for recruiting, an HR department inputs the current employee information so that the algorithm can construct ideal-candidate profiles that are coherent with the company’s values and spirit. This is used in the hope of reducing the errors in hiring someone whose profile does not correspond with the company’s ethos. As a result, the algorithm will favor applicants who are closer to what it considers the best profiles, and therefore, considerably reduce the diversity of applicants. Naturally, this will have an impact on the variety of these applicants, in terms of experience, skills, and personality.
The risk of not seeing on-target profiles
This “carbon-copy” phenomenon not only creates a diminished variety within the company but also tends to dismiss the atypical profiles whose CVs do not check the boxes correctly. A candidate who has followed an unusual career path will have little luck getting through the initial filtering if the algorithm is basing its calculations only on inflexible parameters, thus potentially depriving the company of a high-potential individual. The people who are most likely to experience this are those in the process of changing careers. And the opposite can also occur: A profile that checks all the boxes can make the cut, yet the applicant’s values may not align with those of the company.
Although promising, algorithms are far from being ready to completely replace human recruiters. Even in terms of AI, predictive analytics remain just a tool programmed and configured by a human. If this human lacks goodwill or forethought, an algorithm could easily discriminate at many different levels. On the other hand, if an algorithm is created correctly, it could be invaluable for the first phases of the application process.
Although job-application algorithms are currently used as “homing devices” by businesses to find the ideal profile among the high volume of résumés received, they have limits when trying to quantify the personality of an individual. When it becomes a question of judging the personal skills of a qualified individual who is looking to be hired for a key job that comes with a very high salary, humans should probably always be involved. In fact, when it comes to detecting any candidate’s soft skills, nothing is better than emotional intelligence, which AI technology is currently unable to implement.
Recruiting has already been turned upside down by predictive analytics, but certain human traits remain the best means for judging the social and emotional qualities of others. Algorithms linger at the level of assistants, taking care of the boring and time-consuming tasks, but it could be that we haven’t seen the last of them, and they will one day be used to collect information from our social networks to form a more complex view of our personalities. Who knows…?
Illustration by MarcelSinge
Translated by Mary Wagonner-Moritz
Follow Welcome to the Jungle on Facebook to see our articles in your feed every day!
More inspiration: Apply effectively
It’s time for inclusive holiday PTO: How can you advocate for it?
As the workforce becomes more diverse, companies should let employees take time for the holidays that matter to them
Sep 19, 2024
Can you apply for two jobs at the same company?
Interested in applying for two different jobs in the same company? Here's advice from an HR manager & a dual applicant regarding double applications.
Aug 16, 2022
A trip down memory lane: the evolution of the job hunt from 1970 to 2022
The job hunt and interview process have changed drastically in the last 50 years. Workers and career counselors share their experiences.
Jun 27, 2022
How to break through the overqualification ceiling
An applicant who appears overqualified for a job can put off a potential employer, unless they take simple steps to reassure a recruiter.
Jun 21, 2022
Unsure about applying for jobs in the summer? Just go for it!
Here are 7 tips and reasons behind why you should apply for jobs in the summer.
May 17, 2022
The newsletter that does the job
Want to keep up with the latest articles? Twice a week you can receive stories, jobs, and tips in your inbox.
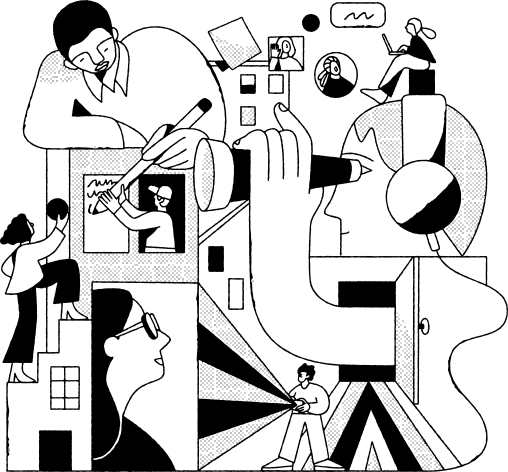
Looking for your next job?
Over 200,000 people have found a job with Welcome to the Jungle.
Explore jobs